Episodes
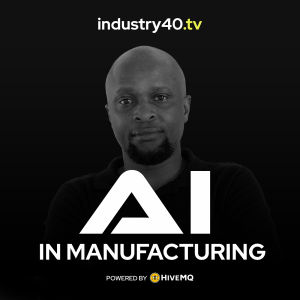
18 hours ago
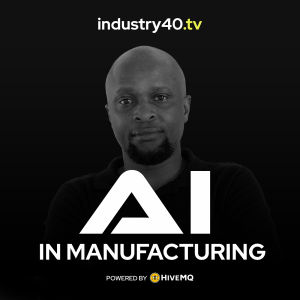
Wednesday Nov 13, 2024
Wednesday Nov 13, 2024
In this episode, we explore how artificial intelligence is transforming manufacturing from the ground up. We dive into cutting-edge applications and discuss the benefits and challenges AI introduces to the industry.
Here’s a sneak peek at what we cover:
1. Predictive Maintenance for Machinery
- AI helps manufacturers predict equipment failures before they happen, reducing downtime and saving costs. With predictive maintenance, companies can transition from reactive to proactive maintenance, leading to longer machine life and fewer unexpected breakdowns.
2. Quality Control and Defect Detection
- AI-powered visual inspections now identify defects faster and more accurately than human inspectors, ensuring product consistency and quality. We discuss how AI-driven quality control is reducing waste and improving overall customer satisfaction.
3. Supply Chain Optimization
- AI tools are optimizing supply chains by predicting demand and adjusting inventory accordingly. In the episode, we break down how smarter supply chains are helping manufacturers avoid bottlenecks and reduce delays, especially during unpredictable market conditions.
4. Enhanced Worker Safety
- From monitoring working conditions to analyzing data for potential hazards, AI is making factory floors safer. Learn how wearable technology and smart sensors are helping manufacturers reduce workplace injuries and improve employee well-being.
5. Energy Efficiency and Sustainability
- AI is enabling manufacturers to cut down on energy usage and reduce their environmental footprint. This is a critical step as companies aim to meet sustainability goals and reduce costs.
🎧 Tune in to the full episode to discover how AI is reshaping the future of manufacturing—and what it means for businesses aiming to stay competitive.
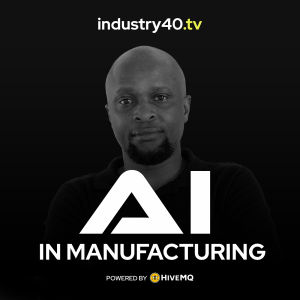
Wednesday Nov 06, 2024
Wednesday Nov 06, 2024
While large language models hold immense potential, there's a significant gap between what these tools offer out of the box and what the manufacturing industry needs.
Manufacturing presents unique challenges that generic AI solutions often can't effectively address.
However, by customizing Generative AI systems to meet industry-specific requirements, this gap can be effectively bridged:
- Tailoring AI to understand specialized language and scenarios enhances its relevance and effectiveness.
- Integrating additional data sources, such as knowledge graphs, enriches the AI's understanding of relationships and processes unique to manufacturing.
- Implementing safety checks and operational boundaries ensures that AI recommendations are viable, safe, and compliant with industry standards.
When these measures are in place, Generative AI becomes a powerful tool applicable to a wide range of use cases.
Tune in to the full episode with Vlad Larichev, the Industrial AI Lead at Accenture Industry X to learn more about Generative AI Use Cases in Engineering and Manufacturing.
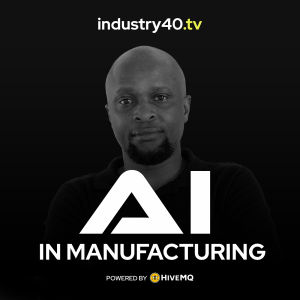
Wednesday Oct 30, 2024
Wednesday Oct 30, 2024
In this episode, I sat down with Michael Kuehne-Schlinkert, CEO of Katulu to discuss how Federated Machine Learning is transforming industrial AI.
Here are some key takeaways:
Federated Learning Enables Cross-Factory Collaboration
Federated learning allows multiple factories to improve AI models without sharing sensitive data. By exchanging learnings, factories can build more robust models while maintaining data privacy and compliance.
Collaboration on Model Training Without Compromising Privacy
One of the biggest challenges in industrial AI is accessing the right data without compromising privacy. Federated learning addresses this by keeping sensitive data local, allowing companies to enhance their AI models collectively without exposing each other’s proprietary or sensitive information.
Cost-Effective Scaling of AI Models Through Reuse
Scaling AI across multiple factories typically involves high costs and complexity. Federated learning significantly reduces development, integration, and operation costs by allowing the reuse of models across different sites without duplicating efforts.
Steamlined Development of Predictive Maintenance and Quality Control Models
Federated learning helps streamline the development of ML models for predictive maintenance and quality control by aggregating insights from multiple sites, reducing the need for extensive data science expertise and making advanced AI accessible to more organizations
Curious about how federated learning can scale industrial AI? Tune in to the full episode to learn more!
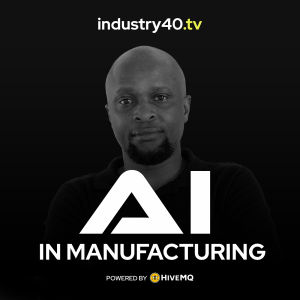
Wednesday Oct 23, 2024
Wednesday Oct 23, 2024
In the latest episode of the AI in Manufacturing podcast on Industry 4.0 TV, host Kudzai Manditereza sits down with Ashan Posohi, CEO and co-founder of Third Wave Automation, to explore how AI-powered robots are transforming material handling. The focus is on autonomous forklifts and their impact on productivity, safety, and the future of manufacturing.
Arshan Poursohi brings a rich background in robotics and research, having worked with Sun Microsystems, Google Research, and Toyota Research. His experiences exposed him to global labor challenges, such as aging workforces and a shortage of young people entering physically demanding jobs. Recognizing the urgent need to address these issues, Arshan founded Third Wave Automation to apply modern AI and robotics solutions to material handling in warehouses and manufacturing environments.
The Business Benefits of AI-Powered Robots
The discussion highlights how AI and robotics are revolutionizing the manufacturing industry by automating "dull, dirty, and dangerous" tasks.
Key benefits include:
- Increased Productivity: Operators can manage an entire fleet of autonomous forklifts from a single workstation, significantly boosting the number of pallets moved per day.
- Enhanced Safety: By removing operators from hazardous environments, the risk of workplace accidents decreases.
- Immediate ROI: Third Wave Automation's as-a-service model allows companies to see immediate returns, with predictable uptime and reduced labor costs.
Shared Autonomy: A Unique Approach
Third Wave Automation introduces the concept of shared autonomy or human-in-the-loop machine learning. Unlike fully autonomous systems that aim for "lights out" operations, this approach keeps humans in the loop:
- Collaborative Operations: Robots perform tasks but can recognize when human intervention is needed, such as when a payload is precariously positioned.
- Continuous Learning: Human inputs help train the AI models in real-time, improving performance and adapting to new scenarios.
- User-Friendly Interface: Operators control robots using familiar tools like steering wheels and screens, making the system accessible even to those without advanced technical skills.
Real-World Impact: A Compelling Case Study
Arshan shares a success story involving a customer who deals with large bags of powder prone to shifting—a challenge for automation due to safety concerns. Using shared autonomy, the autonomous forklifts learned to handle these unstable payloads safely and efficiently:
- Adaptive Learning: The AI models quickly adapted to recognize when human assistance was needed.
- Safety Assurance: Operators could intervene remotely, ensuring safe handling without halting operations.
- Productivity Gains: The customer saw immediate improvements in efficiency and safety, validating the effectiveness of Third Wave Automation's approach.
Listen to the full episode to learn more.
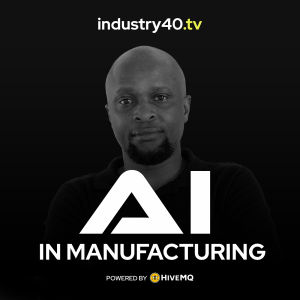
Wednesday Oct 16, 2024
Wednesday Oct 16, 2024
In this episode, I sat down with Yousef Mohassab, CEO of Facilis.ai, to explore how Agentic AI is transforming the manufacturing industry. If you're looking for practical insights on scaling AI and boosting operational efficiency, this is the episode you can't miss!
Here are the key takeaways:
The Shift from Centralized to Agentic AI Manufacturers can no longer afford to rely on centralized data science teams that create bottlenecks. Agentic AI empowers subject matter experts (SMEs) to directly access and analyze data, significantly cutting down response times from weeks to minutes.
A Human-Level Automation Approach Agentic AI mirrors human problem-solving by breaking down complex challenges into smaller tasks. This approach enables real-time, adaptive solutions that evolve with the operational landscape, especially when processes change or equipment gets upgraded.
Beyond Traditional Machine Learning Unlike static models that rely on historical data, self-adaptive systems continuously learn in real-time. This makes AI solutions more relevant to the current operational environment, minimizing the need for human intervention.
Real-World Applications and Results Youssf shared compelling use cases where agentic AI is deployed for quality monitoring, helping companies address shifts in product metrics faster and more effectively. Whether it's vibration analytics or yield optimization, the results are astonishing.
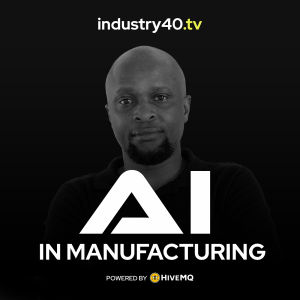
Wednesday Oct 09, 2024
Wednesday Oct 09, 2024
In this episode, I had the pleasure of interviewing Jonathan Wise, Chief Technology Architect at CESMII (Smart Manufacturing Institute).
We discussed how you can modernize your industrial data architecture to harness the full potential of AI, enhancing both production efficiency and innovation.
Jonathan highlighted three key pillars essential for AI readiness:
Data Accessibility - You can’t train AI without accessible data. Jonathan explains why ensuring your data flows seamlessly across systems is the first critical step.
Data Contextualization - Simply having data isn’t enough. Meaningful, contextualized data is crucial for any AI project to deliver accurate and actionable insights.
Data Relationships - It’s not just about isolated data points. AI thrives on the connections between data points, much like how your operations depend on the synergy between suppliers and internal systems.
Listen to the episode to learn more.
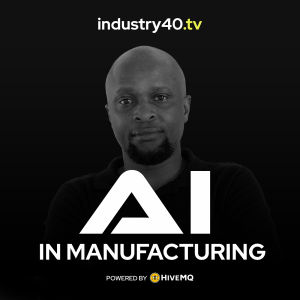
Wednesday Oct 02, 2024
Wednesday Oct 02, 2024
In this episode, we dive deep into the world of smart manufacturing with industry expert Nikunj Mehta from Falkonry. If you're curious about how data is transforming industrial operations and the future of maintenance and reliability, this episode is for you!
Here are some key takeaways:
82% of Failures Are Random
Nikunj explains that a staggering 82% of failures in industrial systems appear random. Without understanding their causes, manufacturers struggle to prevent them. This is where smart, data-driven actions come into play to improve decision-making and reduce failures.
Condition-Based Actions: A Game Changer
In manufacturing, decisions often rely on experience, which can take years to accumulate. Condition-based actions allow manufacturers to make smarter decisions without needing decades of experience. By detecting and acting on real-time conditions, manufacturers can optimize maintenance, improve quality, and reduce emissions.
Real-Time Data = Real-Time Decisions
From mining to steel production, the power of real-time data can revolutionize how we handle variations in materials, weather conditions, and equipment performance. Nikunj shares how timely insights enable proactive decision-making, reducing downtime and energy waste.
Smart Guidance Systems
Smart manufacturing requires systems that can analyze data in real-time and offer actionable guidance. Think of it like a GPS for your factory: these systems navigate complex production challenges and direct optimal actions for maintenance, quality control, and emissions.
What's Next for Smart Manufacturing?
Nikunj forecasts that the next step in manufacturing will be integrating smart guidance systems across various processes—from maintenance to quality assurance—allowing companies to move from reactive to proactive management.
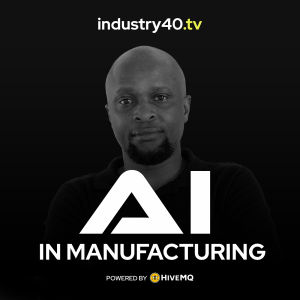
Wednesday Sep 25, 2024
Visual Intelligence Applications in Manufacturing: Cyrus Shaoul - CEO, Leela AI
Wednesday Sep 25, 2024
Wednesday Sep 25, 2024
In our latest podcast episode, I had the pleasure of speaking with Cyrus Shaoul, CEO of Leela AI, about visual intelligence and its transformative impact on manufacturing operations.
Here are some Key Takeaways:
1️⃣ Beyond Traditional Machine Vision
Unlike traditional machine vision systems that focus on product inspection, visual intelligence looks at the entire manufacturing process. It helps identify value-adding activities in real-time, ensuring operational excellence is met consistently.
2️⃣ Uncover Hidden Performance Insights
By integrating visual intelligence, companies can detect bottlenecks and wasted time during manual operations. In one case, Lila AI improved line capacity by 20% by identifying areas where standard operating procedures weren’t being followed.
3️⃣ Boost Safety & Compliance
With advanced monitoring, manufacturers can significantly reduce safety violations. One customer saw a 50% reduction in non-compliant events, leading to fewer accidents and a safer work environment.
4️⃣ Improving Quality Control
Visual intelligence doesn’t just ensure processes run smoothly; it improves quality control by catching invisible defects in real-time, boosting yields by 10%. This kind of proactive monitoring helps prevent costly mistakes that traditional methods might miss.
5️⃣ Faster, Data-Driven Decisions
With visual intelligence, data is constantly collected and analyzed, allowing teams to make real-time adjustments and enhance productivity, safety, and quality simultaneously. The ROI on this technology speaks for itself.
🎧 Tune in to hear the full conversation and explore how visual intelligence is reshaping the future of manufacturing.
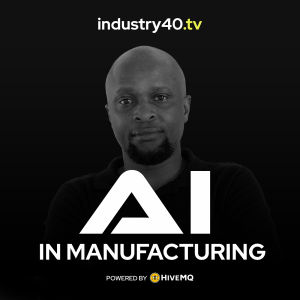
Wednesday Sep 18, 2024
Wednesday Sep 18, 2024
In my latest AI in Manufacturing podcast episode, I had the pleasure of interviewing Peter, CEO of XMPRO where we discussed How to Build Intelligent Digital Twins with Generative AI.
Here are five key takeaways: